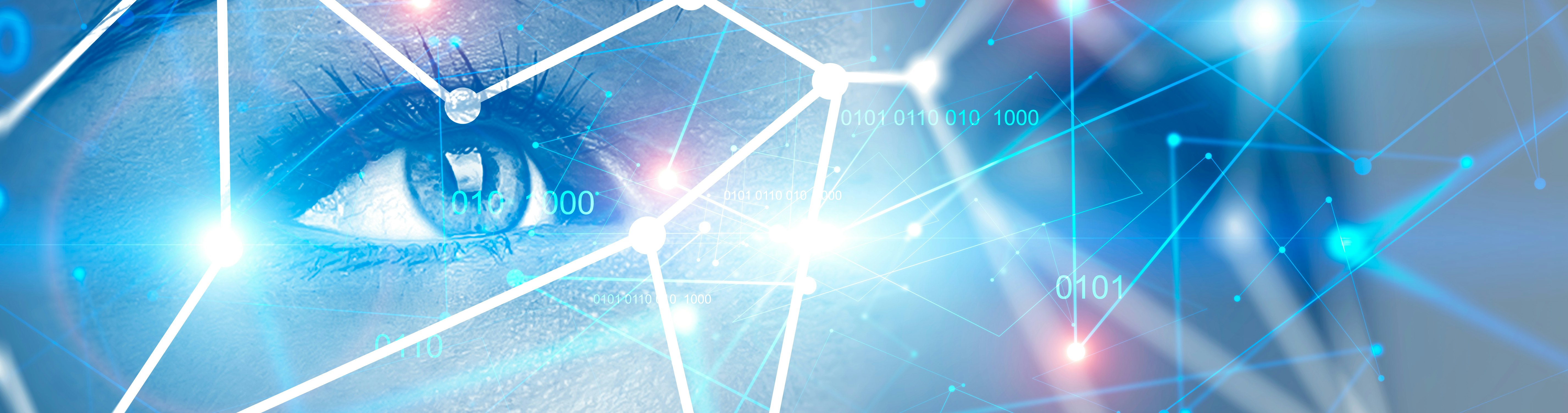
Is it Data Lake vs. Data Mesh? Or can they complement each other?
In recent years, the exponential growth of data has led to an increasing demand for more effective ways to manage it. Building a data-driven business remains one of the top strategic goals of many business stakeholders. And while it may seem logical for companies to embrace the idea of being data-driven, it’s far more difficult to execute on that idea.
Data Mesh and Data Lakes are two important concepts in the world of data architectures that can work together to provide a flexible and scalable approach to data management. Data Lakes have already proven to be a popular solution, but a newer approach, Data Mesh, is gaining attention. This blog will dive into the two concepts and explore how they can complement each other.
Data Lakes
A data lake is a large and central storage repository that holds massive amounts of data, from various sources, and in various data formats. It can store structured, semi-structured, and unstructured data (e.g. images).
Think of it as a huge pool of water, where you can store all sorts of data, such as customer data, transaction data, social media feeds, images, videos and more. It is a cost-effective and accessible solution for companies dealing with large data volumes and various data formats.
Additionally, data lakes allow teams to work with raw data, without the need for extensive preprocessing or normalization.
Data Mesh
Data Mesh is a relatively new concept that takes a decentralized approach to data management. It treats data as a product and is managed by autonomous teams that are responsible for a particular domain.
Data Mesh advocates that data should be owned and managed by the people who understand it best - the domain experts - and should be treated as a product. It means that each team is responsible for the data quality, reliability and accessibility of data within its domain.
This creates a more scalable and flexible approach to data management, where teams can make decisions about their data independently, without requiring intervention from a centralized data team.
How can data lake technology be used in a data mesh approach?
In short, Data Mesh is an architecture where data is owned and managed by individual product teams, creating a decentralized approach to data management. A data lake is a technology that provides a centralized storage solution, allowing teams to store and manage large amounts of data without worrying about data structure or format.
Decentralization in Data Mesh is about taking ownership of sharing data as products in a decentralized way. It’s not about abandoning centralized storage solutions, such as Data Lakes, but about using them in a way that adheres to the principles of Data Mesh.
Data Mesh is all about defining and managing Data Products as a building block to make data easily accessible and reusable for various use cases. Each ‘Data Product’ should be able to provide its data in multiple ways through different output ports.
An output port is aimed at making data natively accessible for a specific use case. Example use cases are analytics and reporting, machine learning, real-time processing, etc. As such, multiple types of output ports need corresponding data technologies that enable a specific access mode.
One technology that can support a Data Mesh architecture is a data lake. The data in an output port for a data product can be stored in a data lake. This type of output port then receives all the benefits offered by data lake technology.
In a Data Mesh architecture, each data product gets its own segment in the data lake (e.g. an S3 Bucket). This segment acts as the output port for the data product, where the team responsible for the data product can write their data to the lake. By segmenting the data lake in this way, teams can manage and secure their own data without worrying about conflicting with other teams. As such, decentralized ownership is made possible, even when using a more centralized storage technology.
While a data lake is an important technology for supporting a Data Mesh architecture, it may not be the ideal solution for every use case. Using a data lake as the only type of data storage technology may limit the flexibility of the Data Mesh platform, as it only provides one type of storage. For example, when it comes to business intelligence and reporting, a data warehouse technology with tabular storage may be more suitable. Another example is when time series databases or graph databases are a better option because of the type of data we want to make natively reusable.
To make the Data Mesh platform more flexible, it should provide the capability to plug in different types of data storage technology. Each of them is a different type of output port. In this way, each data product can have its own output ports, with different types of data storage technologies, geared towards specific data usage patterns.
We have noticed that cloud vendors frequently recommend implementing a Data Mesh solution using one of their existing data lake services. Typically, their approach involves defining security boundaries to separate segments within these services, which can be owned by different domain teams to create various data products.
However, the reference architectures they provide only incorporate one storage technology, namely their own data lake technology. Consequently, the resulting Data Mesh platform is less adaptable and tied to a single technology. What is lacking is an explicit ‘Data Product’ abstraction that goes beyond merely enforcing security boundaries and allows for the integration of various data storage technologies and solutions.
Conclusion
Data management is a critical component of any organization. Various technologies and approaches are available, like data lakes, data warehouses, data vaults, time series databases, graph databases, etc. They all have their unique strengths and limitations.
Ultimately, a successful Data Mesh architecture provides the flexibility to share and reuse data with the right technology for the right use case. While a data lake is a powerful tool for managing raw data, it may not be the best solution for all types of data usage.
By considering different types of data storage technologies, teams can choose the solution that best meets their specific needs and optimize their data management workflows. By using data products in a Data Mesh, teams can create a flexible and scalable architecture that can adapt to changing data management needs.