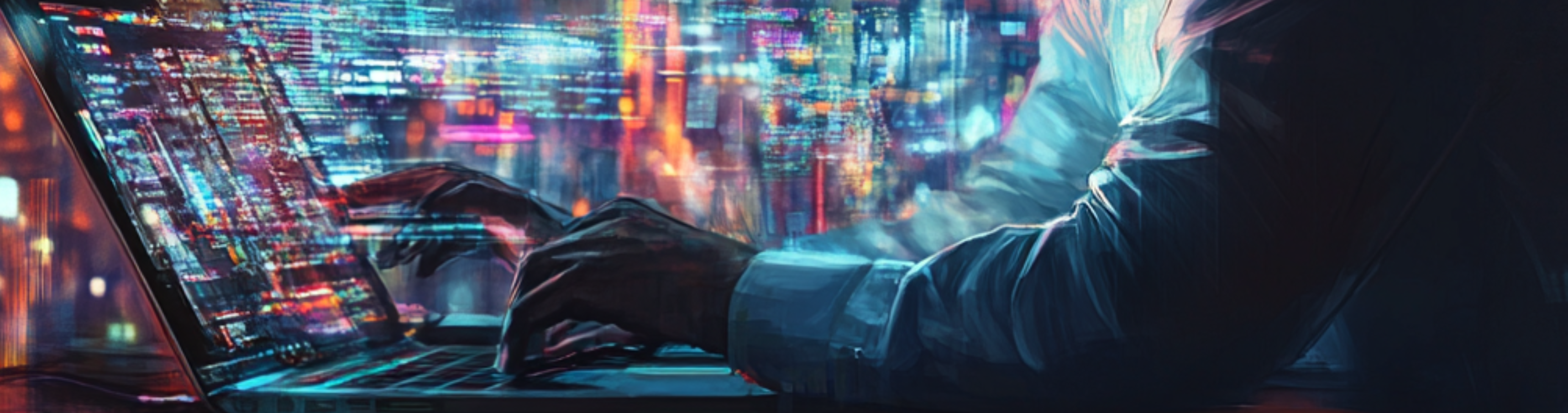
How AI is changing data analysis (and what you need to know as a data analyst)
The world of data analysis is changing fast. AI tools like Copilot are automating tasks that used to take us hours, which is exciting! But it also means we need to evolve our skills to stay ahead of the curve. Instead of spending time on repetitive tasks, data analysts can now focus on the bigger picture: strategy, problem-solving, and truly understanding the business. This blog explores the key skills data analysts need to thrive in this new AI-powered environment.
The data analyst’s new focus: from repetitive tasks to strategy
Imagine having more time to focus on what really matters: understanding the business, solving complex problems, and making strategic decisions. That's the opportunity AI provides. To maximize Copilot’s potential, data analysts need to shift their focus from manual tasks to work that require deep business knowledge and critical thinking.
A crucial part of this shift is collaborating closely with stakeholders. Data analysts need to understand their challenges, define the right questions, and ensure their insights truly drive decision-making.
Key skills data analysts need when working with AI
1. Advanced data modeling and metadata management
Why it matters: With AI tools like Copilot handling much of the front-end report creation, the quality of insights will increasingly depend on the robustness of the underlying data model. Data analysts should invest time in refining their data modeling and metadata management skills.
Actionable steps:
- Ensure that data models are clean, scalable, and well-documented. Be honest, how often have you filled out the “Description” field in your Power BI data model? How often have you used the “Synonyms” field? Our guess is: not all that often. Ironically, these fields will now be crucial in your pursuit of qualitative responses from Copilot … You will need to organize metadata to improve discoverability, ensuring Copilot (or other AI tools) can leverage the right data to generate insights.
- Build a deep understanding of how to structure data to enable AI to create actionable, accurate insights. Take a good hard look at your data model and how it is built. Define what can be improved based on best practices, and then apply them systematically.
2. Data governance and quality assurance
Why it matters: Copilot can only produce reliable outputs with high-quality data. Data analysts will need to focus on ensuring data consistency, reliability, and governance.
Actionable steps:
- Implement and maintain best practices for data governance.
- Use clear naming conventions, predefined measures, and logical data structures that make it easier for Copilot to generate actionable insights.
3. Business acumen and strategic insight generation
Why it matters: AI tools lack contextual understanding, so data analysts must bridge this gap. Developing a strong grasp of business operations, industry trends, and strategic objectives allows analysts to create insights that are both relevant and impactful.
Actionable steps:
- Invest in learning about your organization’s goals and strategic challenges. The clearer you can understand and document these goals and challenges, the better you will be able to translate them into relevant insights.
- Regularly engage with business leaders to understand the context behind the data, which in turn helps translate findings into actionable strategies.
4. Communication and storytelling skills
Why it matters: Translating technical insights into stories that resonate with business stakeholders is crucial. Storytelling bridges the gap between data and decision-makers.
Actionable steps:
- Become an expert at framing the insights. Work on presenting data in narrative formats that highlight the “why” and “how” behind the insights.
- Focus on how the data aligns with the company’s goals, offering clear recommendations and visualizations that stakeholders can easily grasp.
How to implement these skills: practical actions for data analysts
Developing data modeling and metadata management skills
With AI tools like Copilot in the mix, the quality of insights depends significantly on data models. Data analysts should dedicate time to refining their data modeling skills, focusing on:
- Organizing and documenting data: Pay attention to metadata fields like descriptions and synonyms, which will help AI generate more accurate outputs.
- Data structure optimization: Ensure your data structure is scalable, clean, and flexible. This will streamline Copilot’s ability to work with the data seamlessly.
Engaging with business stakeholders
AI-generated insights are only as valuable as their alignment with business goals. Data analysts must regularly engage with stakeholders to:
- Define clear objectives: Discuss goals and pain points with stakeholders to set a clear direction for AI analysis.
- Gather feedback: Regular feedback helps adjust AI-generated insights to better meet business needs, ensuring outputs are practical and actionable.
Conclusion: the future of data analysis is here
AI tools like Copilot are transforming data analysis, and it's an exciting time to be in this field! By focusing on strategic thinking, communication, and strong data foundations, data analysts can not only adapt but thrive. The ability to connect data insights to business context, combined with excellent communication and storytelling, will define the most successful data analysts in the years to come.
By investing in these skills, data analysts can stay at the forefront of data-driven innovation. For more insights on how Copilot is shaping data analysis, read the article “How Copilot in Power BI is Transforming Data Analysis”.